Ensuring the credibility of simulation models is a concern in all fields of engineering. In aerospace engineering, structural qualification is a crucial step in ensuring that critical components are capable of withstanding the most severe operational conditions. This process is essential to ensure the safety and reliability of systems during missions. This article focuses on the qualification of the Dual Launch Structure (DLS) of the Ariane 6 launcher, developed by ArianeGroup. This qualification aims not only to validate the structure itself, but also to confirm that the numerical simulation model used can reliably predict the structure’s behavior, thus minimizing dependence on future physical testing.
The aerospace industry relies heavily on simulation models to predict the behavior of complex systems under different conditions. Accurate models can reduce the need for extensive physical testing, saving time and money. Ariane 6, developed by ArianeGroup, is designed to meet the demands of a competitive space market, offering configurations such as Ariane 62 and 64 to accommodate different payloads. One of the central elements of its design is the Dual Launch Structure (DLS), which has to withstand significant stresses during launch.
Ariane 6 aims to provide a versatile, cost-effective solution for space launches. The DLS plays a crucial role in this system, supporting payloads and ensuring structural integrity throughout the launch sequence. Its qualification is therefore an essential stage in the launcher’s development. In parallel, validation of the DLS simulation model must confirm that the model can reliably predict the structure’s performance under various load conditions, minimizing the need for extensive physical testing in future designs.
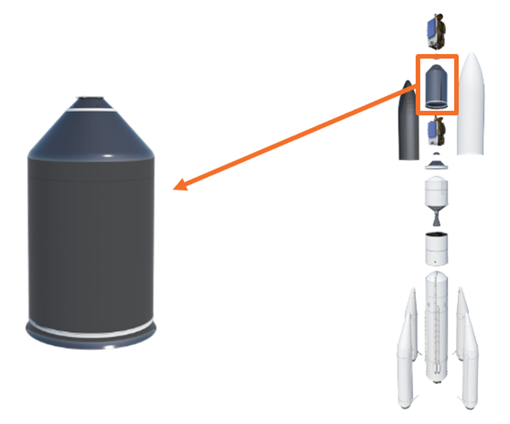
ArianeGroup provided the technical expertise and infrastructure required to carry out the full-scale static tests, which were crucial in assessing the DLS’s response to axial, bending and lateral loads.
The collaboration between EikoSim and ArianeGroup sought to add to the qualification a validation process directly integrating experimental and simulation approaches. In particular, EikoSim contributed its expertise in Digital Image Correlation (DIC), a technique for measuring displacement and deformation on the surface of the instrumented component. This method complements traditional point measurement techniques, offering a more complete view of structural responses.
The qualification process, based on the test pyramid approach, concluded with a scale 1 test at failure, combining several stresses and enabling the real margins of resistance to be defined. First of all, elementary loadings were carried out. For each of these loads (axial, lateral, etc.), the stiffness of the DLS was assessed, along with its ability to withstand the design limit loads.
Instrumentation was an essential aspect of the data collection process. Around 200 strain gauges were placed at strategic locations to measure the structure’s deformation levels. These gauges provided point data on the distribution of strains within the DLS. In addition, some 30 displacement sensors were used, providing information on global deformation modes. Rayleigh backscattering fiber optic sensors were also used to provide continuous data on deformation along the fibers, improving the resolution and accuracy of deformation measurements.
Finally, digital image correlation (DIC) played an essential role in the validation process. Three DIC systems were deployed in the crucial regions identified by preliminary FEM analyses, complemented by marker-tracking measurements.
A data fusion environment was also developed by EikoSim to integrate complementary measurement data. Comparison between FEM predictions and experimental data revealed a high degree of correlation, with deviations generally within acceptable limits.
For example, strain measurements in critical areas showed deviations from predicted values of less than 20%, which is considered acceptable at this step for structural validation purposes. Analysis of these deviations revealed that they were mainly due to simplifications in the model or minor inaccuracies in the measurement device. For example, nominal (non-registered) boundary conditions and slight differences between the material properties assumed in the model and the actual properties could explain some of the discrepancies observed in the deformation data.
The agreement between the finite element model and the experimental results reinforced the credibility of the simulation, affirming its relevance for future predictive analyses. This validation also served as a basis for refining the model, for example by adjusting the boundary conditions to better align with the observed data.
The project illustrated the concept of “Smart Testing”, in which simulation and physical testing are not only complementary, but mutually reinforcing. Preliminary simulations guided the design of the test fixture, ensuring that critical load paths and potential failure points were adequately monitored. The physical test fixture was optimized on the basis of the results of the finite element method, approximating the expected operational conditions for the DLS. The integration of DIC data with traditional measurement techniques provided a comprehensive view of DLS behavior, boosting confidence in the simulation results.
In the future, the focus will be on refining the FEM validation process, including the development of more sophisticated validation measurements. These measures will enable more precise quantification of the simulation model credibility and identification of specific areas for improvement.
In addition, efforts will be directed towards the implementation of automated model optimization processes, which can adjust model parameters on the basis of experimental data, further improving model accuracy and reliability.